Sahir Bhatnagar
Biostatistician, Statistical Geneticist, Associate Director, Adjunct Professor
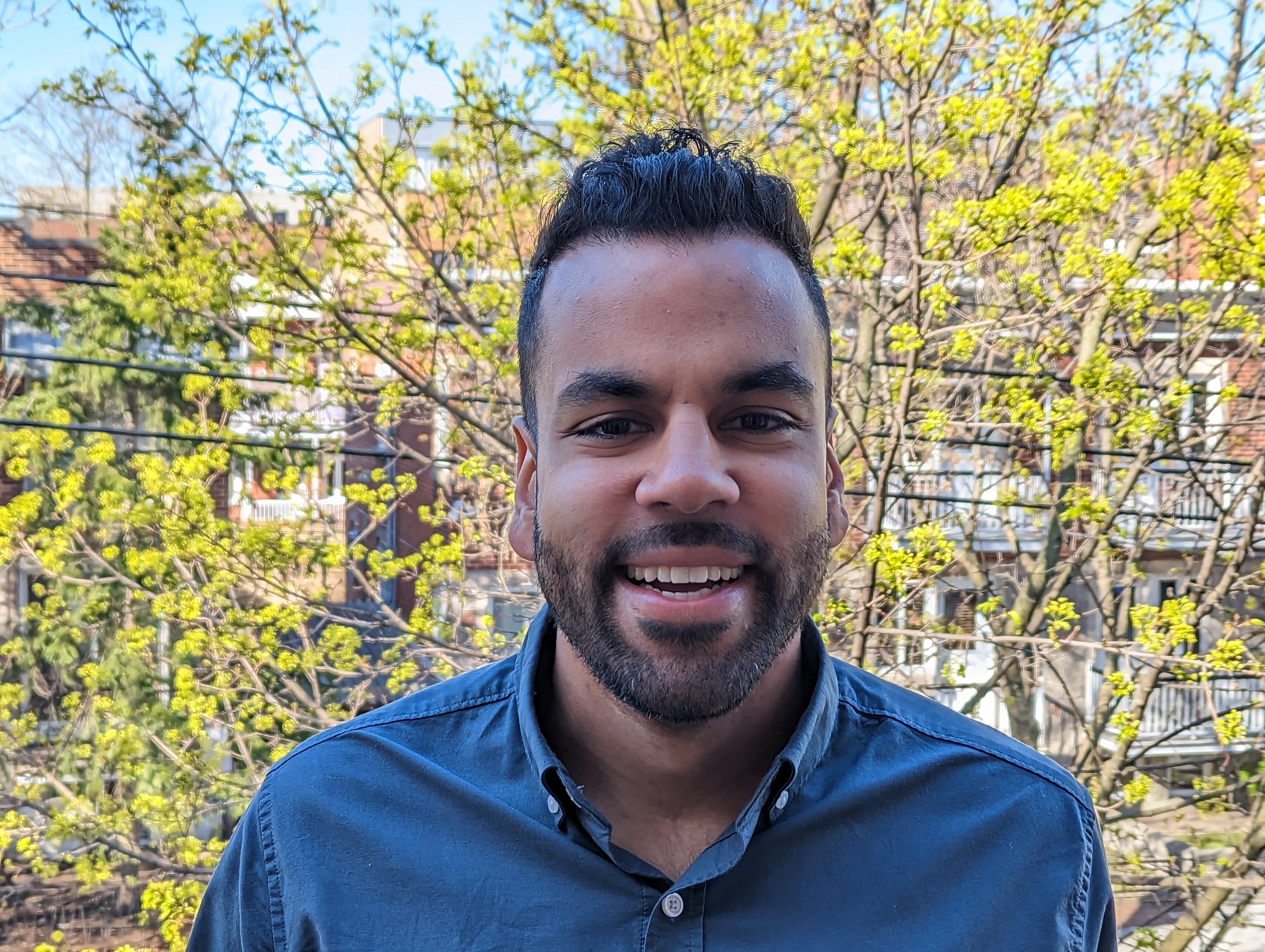
Welcome to my website. I’m Sahir, a biostatistician who uses statistical methods to answer questions that are important to clinicians. After obtaining my PhD in Biostatistics, I joined McGill University as an Assistant Professor in the Departments of Epidemiology, Biostatistics, and Occupational Health, and Diagnostic Radiology. My research has focused on developing statistical methods for analyzing high-dimensional data in genomics and radiomics. I’ve published in journals such as Nature Genetics, PLoS Medicine, Biometrics, Bioinformatics, Statistics and Computing, PLoS Genetics, Computational Statistics and Data Analysis, the Journal of the Royal Statistical Society Series C, and the Journal of Statistics and Data Science Education. I’m grateful to have been funded by an NSERC Discovery grant with launch supplement and several CIHR Project Grants.
I’m also the author of several R packages that have been downloaded over 350,000 times. The most rewarding part of my job has been training and supervising students. I’ve also taught a Masters level course in Biostatistics for 4 years with an average enrollment of 100 students and several short courses on statistics and machine learning.
I then transitioned to Deep Genomics, as a Senior Research Scientist on the Target Identification team. My work focused on the identifying new drug targets using whole genome sequencing data. Currently, as the Associate Director of Statistical Genetics at 5 Prime Sciences, I provide large pharmaceutical partners with human genetics evidence to de-risk their drug development pipelines.
Feel free to reach out if you have any questions about my work, or if you would like to collaborate. While I am no longer taking on graduate students, I maintain a connection to academia as an Adjunct Professor at McGill.